What Is AI TRiSM, and Why Is it Time to Care?
Fearing renegade AI projects in user departments or applications tainted by flawed data, organizations are looking for a bit of structure in their AI initiatives.
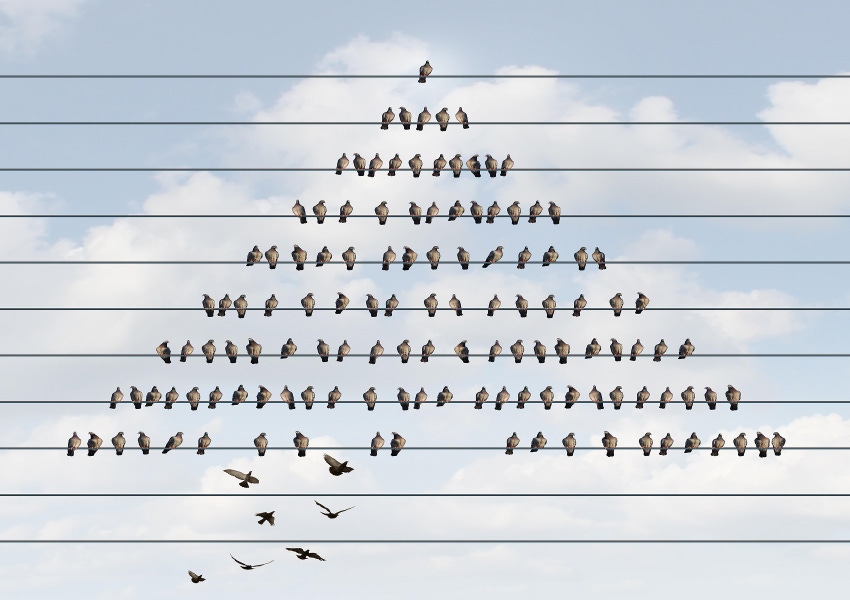
Artificial intelligence trust, risk, and security management (AI TRiSM) is a technology and policy framework for managing AI. It’s gaining traction in enterprises as they grapple with the questions of how, when and where to best use AI, while also keeping the AI compliant and reliable.
This is not an easy task.
The Challenges of AI Adoption
Short on AI skills, many organizations begin their AI journeys by relying on vendors and outside business partners to provide turnkey AI solutions. These systems manage IT operations, financial and healthcare databases, weather forecasts, website chat sessions, and other functions.
For many companies, this strategy of outsourcing AI isn’t likely to change, because they don’t have the in-house expertise or the financial resources to invest in AI on their own. For other companies where AI is both strategic and affordable, they need to trust the goodness of their own AI models, data and results.
In both cases, there is a responsibility for AI. That means asking key questions: Is the AI trustworthy? What are the risks of using AI if it fails? Is the AI secure?
The Central Role of AI Trust, Risk, and Security Management
The goal of AI TRiSM is to place the necessary trust, risk and security guardrails around AI systems so that enterprises can ensure that these systems are accurate, secure and compliant.
This can be a daunting undertaking, for while there are many years of governance experience and best practices for traditional applications and structured system of records data, there are few established best practices when it comes to managing and analyzing AI structured and unstructured data, and their applications, algorithms and machine learning.
How, for instance, do you vet all of the incoming volumes of data from research papers all over the world that your AI might be analyzing in an effort to develop a new drug? Or how can you ensure that you are screening databases for the best job candidates if you are only using your company’s past hiring history as your reference?
Let's Take a Closer Look at the AI TRiSM Framework
AI TRiSM addresses these questions with a framework for managing AI data and systems. This framework includes the following four elements:
AI Explainability
If you use AI to obtain results and then draft a report to the board, do you feel secure when someone asks you how you arrived at your conclusion and what data sources were used?
It’s a big question that encountered the school of hard knocks in 2018, when Amazon de-implemented an in-house AI recruiting system that disproportionately favored male over female job applicants.
Upon closer examination, the company realized that the only data it had fed to the AI system was from its own internal employment database. That data showed that in past years, the company had hired more males than females. Consequently, the company missed out on a large pool of qualified female applicants.
Next Steps for Implementing AI Explainability
No one wants to stand in front of their board trying to explain how AI went wrong. So, a best practice is to assure that you have a broad enough data source base for your AI before you run it. Equally important is that you cross-check your AI queries and algorithms to ensure that they are as inclusive and non-biased as possible.
The ultimate test is within yourself. If asked, can you confidently explain to yourself how the AI derived its conclusions, and what data it used to arrive at results?
AI Model Operations
After deployment, AI must be maintained. The challenge for enterprises is how to maintain it.
With systems of records, you perform maintenance by continuously monitoring performance and fine-tuning as needed, and by resolving software bugs when they occur. This form of maintenance has a running history of over 70 years, so there is no confusion about how to perform it.
In contrast, AI systems have few established maintenance practices. When AI is first deployed, it’s checked against what subject matter experts in the field would conclude, and it must agree with what these experts conclude 95% of the time.
Over time, business, environmental, political and market conditions change. The AI application (and its conclusions) must change with them. This is where AI maintenance comes in.
Next Steps for Implementing AI Maintenance
If an AI system’s outcomes suddenly decline in accuracy from 95% to 85%, it’s time for a cross-disciplinary team of user subject matter experts and IT/data science to get together to see if data sources, algorithms, machine learning, etc., must be fine-tuned so they can re-align with the business and get back to the level of accuracy that the enterprise expects.
If AI maintenance isn’t regularly performed, AI system results will lose accuracy. This increases company risk because the outcomes that management decisions are based upon could be wrong. Incorporating the AI TRiSM framework is designed check this.
AI Security
Hackers are finding new ways to attack AI systems as deployments grow.
One line of attack is data poisoning. This happens when a hacker gains access to AI model training data and corrupts the data, so the system produces erroneous results.
In AI security, AI TRiSM assumes that traditional IT methods of AI security enforcement are already in place. These include setting high authentication standards for AI users that include multi-factor authorization, building zero-trust networks and network segment boundaries around AI systems that further constrain access, and securing hardware, operating systems and applications. However, a second tier of AI security enforcement is also needed, which AI TRiSM addresses.
Next Steps for Implementing AI Maintenance
This second security front involves the vetting of all data source providers for AI projects. The goal is to ensure that each provider meets or exceeds enterprise governance and security standards. IT should use additional data preparation tools to ensure that all data entering into an AI data repository has been cleaned, formatted and properly prepared for use.
AI Privacy
Enterprises have their own data privacy policies, but with AI, it’s also necessary to ensure that the data brokers whom you purchase data from have similar data privacy policies. Focusing on this is core to the AI TRiSM framework.
For example, a large healthcare lab wanted to study the impact of cancer treatments on a variety of patients from Europe. It contracted with a number of hospitals and clinics to obtain patient data. The stipulation made to each data provider was that all patient health data was to be anonymized so that patient privacy was protected.
The lab obtained the variegated data from hospitals and clinics that it had asked for, and the data could be analyzed by geographical location, type of cancer, age and gender. However, there were no individual patient identifiers present in the data. All data providers had anonymized patient records to protect the privacy of patients.
The Future of AI TRiSM
Gartner says that by 2026, 80% of companies will be using technologies like generative AI, but few enterprises have inked the words “AI TRiSM” on their IT strategic roadmaps.
The good news is that many companies are already performing the steps that AI TRiSM enumerates. Enterprises are designing tightly orchestrated use cases where AI can benefit the business. They are actively deploying security measures like zero-trust networks and are doing a better job of vetting data brokers for security and data privacy compliance.
One lagging area is AI system maintenance and fine-tuning, but this is likely to garner more attention as more AI gets deployed.
Meanwhile, CIOs should start thinking ahead about what their IT audit and regulatory checklists are likely to look like in five years. You can expect auditors and regulators to come in with AI TRiSM checklists, and IT must be ready to check off all the boxes.
About the Author(s)
You May Also Like