Ripples of Innovation and New Business Needs from GenAI's Splash
Generative AI may have set off a cascade of additional resources needed to sort out how to best take advantage of this technology.
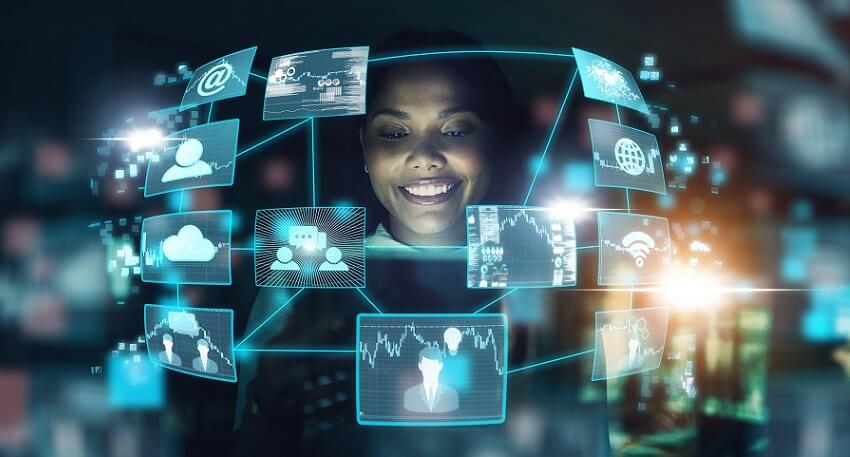
Soon after OpenAI’s ChatGPT lit the bonfire for generative AI (GenAI), many companies raced to introduce solutions, apps, and platforms that leveraged such technology. The phrase “powered by AI” soon became not only a marketing strategy but an operational goal for organizations that want a share of the spotlight and fear the competitive use of AI might leave them behind.
Promises of improved efficiency through such automation, freeing up staff to tackle other work -- as well as the potential for some job elimination -- became part of the expectations for this new era of AI. Whether bolted on to existing resources or new innovations built from the ground up around GenAI, companies became eager to show they could put the technology to work.
GenAI’s impact also set off ripples across ancillary hardware and software sectors that sought to respond to new needs and questions that arose in relation to the technology. Where are the chips that can support AI in devices? What kind of oversight and observability is needed in the use of GenAI? Is there room for newcomers or will incumbents dominate the data and resources that support AI?
A host of stakeholders offer a barometer of what innovations and services are emerging in response to GenAI and demands this space may face as it proliferates and spreads.
Ethics and Investment in AI
Investment into AI ramped up fast with the likes of Accenture committing to extensive backing of the space. “There was an arms race to say how much they were investing,” says David Cushman, executive research leader with HFS Research. A massive explosion of announcements in 2023, he says, came from companies doing deals to demonstrate how they meant to play in this arena. Last summer, Accenture declared its plans to invest $3 billion and to double its AI staff from 40,000 to 80,000 globally.
“Across the whole market, we saw 50, 60, 70 [press] releases in three months of people saying, ‘We’ve done a deal with Google; we’ve done deal with Microsoft; we’ve done a deal with OpenAI,’ with just such massive excitement about it,” Cushman says. He compares the possibly historic spread of this technology, growing so far so quickly, with Web 2.0.
Among other areas, Cushman sees a wave of new businesses emerging that use natural language to build apps, such as Bitmagic and AI21 Labs. “Bitmagic you can use text to create a game -- prompt a game into existence,” he says. “Those are already with us.” A second wave is coming, Cushman says, where natural language will be used to interact with and train applications, such as Rabbit and its forthcoming R1, AI assistant device. The interaction with AI could become something like a series of digital nesting dolls training each other. “That’s actually using apps to train other apps to create another app,” he says.
A third wave, Cushman says, may include using natural language to create agents, called agentic AI systems, which could operate with little supervision to fulfill complex tasks.
As more companies embrace AI, there may be questions about the technology’s ethics and how it might be governed within an organization, which may lead to a need for help with ethics as a service. “How should AI behave in order to not be biased? How should AI behave in order not to swear at people?” Cushman asks. A response emerging from service providers, he says, is to set up a responsible AI office to monitor legislation and changes coming down the line that could impact this space. Cushman also says some providers might offer responsible AI as a service; however, he questions putting such duties in a third party’s hands, digital or otherwise. “I think you should own your own ethics,” he says. “You certainly shouldn’t expect a machine to learn your ethics. That’s kind of crazy.”
Tools and Data for AI
Part of taking advantage of AI may include building out resources and aligning data that can work with it. “With every seismic shift in technology, a new architecture was born to rise to meet the challenges of that shift,” says Michael Gilfix, chief product and engineering officer at vector database company KX. “With the advances in natural language processing, there’s a whole host of new tools and technologies that have come to bear to solve the end-to-end problem of creating intelligent applications, whether those are ones that we experience through natural language exchange like chat, or they could be intelligence that’s embedded inside applications themselves.”
Vector databases, he says, can make unstructured data searchable and useable by natural language technology.
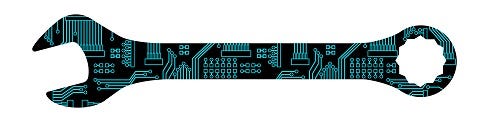
Source: Byfotohansel via Adobe Stock
Gilfix says there are innovations in vector databases such as coming up with intelligent forms of search to help find the best quality information. “It isn’t just sufficient to match the unstructured data using something called similarity search,” he says. “You also need to relate it to structured data as well … so in many cases people are advancing their search algorithms so that they’re really smart about finding data.”
For example, if something gets misspelled, the system can still figure out that is the actual data that is of interest. “There’s advanced scoring algorithms that are emerging to ensure that vector databases find the right things,” Gilfix says. “There’s a lot of other tools that are emerging, so vector databases are sort of a core element of providing the fact base to a natural language model.”
As technologies help fine-tune such models, he says there is also a growing need for a mix of vector databases to serve as the fact basis. “You need fine-tuning when you want to tweak the personality or the domain understanding of your natural language model.”
AI Offering Observability into AI
Knowing what is happening with AI within an organization, and who has access to it, is another aspect of its implementation that leadership might focus on. The concept of observability, often associated with security and resource management, could come into play here.
There are different schools of thought on observability when it comes to AI, says Amol Ajgaonkar, CTO of solutions and system integrator Insight. “One is the operational observability,” he says. “If I’m running a solution and I’m using the foundational model or some generative AI model, a large language model … I’m interested in understanding what is the latency. What is the performance? What is the cost of it? How many hallucinations did I get out of that? Did it just make up stuff? How many times did that happen? Did I capture the user feedback on certain responses?”
Those questions may seem daunting, but with the right resources, he says, it may be possible to sort out the answers. “Metrics and logging and the cost of running the model on-prem versus running it in the cloud versus using a third-party service or a model -- all of those metrics will be captured and logged,” Ajgaonkar says. GenAI might be applied on top of those logged metrics, he says, to ask the right questions to gain further insight.
Audit Services for AI
Putting AI to work can also come with compliance questions as regulated companies may need to adhere to prior and developing policies that relate to the use of the technology. Andrew Pery, AI ethics evangelist with document processing and data capture company ABBYY, says policies such as EU regulation can provide some flexibility with conducting voluntary audits of adherence to laws applied to AI. However, real challenges remain in terms of ensuring there is an objective assessment of compliance. “What we’re seeing is the emergence of this new discipline, which is the independent audit of AI systems,” he says. “Just like you have auditors who are auditing financial performance, we’re going to see a new discipline of subject matter experts whose mission is to conduct objective independent audits of AI systems.”
ForHumanity is an organization Pery says stands at the forefront of this, working with regulators in most jurisdictions around the world. “They’re working with the EU, with the UK GDPR; they're working with US regulators as well as Canadian regulators,” he says. “What they’re doing is developing essentially independent audit criteria that maps into the regulations, the requirements of those regulations, as well as alliance with the standards organizations.”
AI Chipsets
On the device side, AI’s popularity drives an effort to bring such resources from the cloud space to user’s hands. “The novel thing about this innovation cycle is on-device, generative AI,” says Paul Schell, industry analyst with ABI Research. Though AI has been on-device for some time, he says, its earlier iterations dealt with simpler, lighter workloads for such tasks as image enhancement or gaming applications.
“It’s the transformer models that are running on-device,” Schell says. “The sort of trimmed-down, quantized versions of bigger models that used to be constrained to cloud environments that are now able to run on-device on chipsets like, for example, the Snapdragon 8 Gen 3 and the Dimensity 9300 from MediaTek that are sort of unlocking a new wave of productivity applications.” This also applies to the PC market, he says, with the Intel Core Ultra processors that have been released and AMD’s Ryzen AI engine. “This applies to consumer and enterprise markets,” Schell says.
Incumbents in smartphones such as Qualcomm, MediaTek, and Google have been making moves in this space, he says. Meanwhile in the PC market, Intel and AMD as well as ARM-based processors are pursuing opportunities with AI-chipsets.
Though AI resources are available in the cloud, there are some expected benefits with AI chips. “The value proposition of keeping it on device is threefold,” Schell says. “It’s much lower latency … networking costs, and then there’s also the most, I think, crucial one, which is data privacy.”
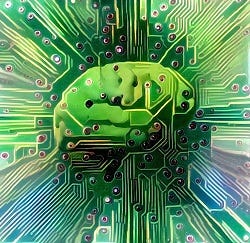
Bruce Rolff via Alamy Stock
That means the AI model running on-device keeps the data on the device. “It’s much more secure that way, and it means that their output and their training can be much more personalized to your own user data,” he says.
AI chipsets could make AI more attractive to industries that have been reluctant to adopt the technology, according to Reece Hayden, senior analyst with ABI Research. “If we look at a couple of verticals like manufacturing or healthcare that have been kind of opposed in the past to deploying AI on at their sites, mainly due to that data privacy risk or that data sovereignty risk, which we’re seeing in Europe at the moment -- when we look at moving that processing from the cloud to the device, this is where you can actually start to see those use cases in manufacturing and healthcare, these very regulated, highly data sensitive verticals actually taking off. ”
That could provide more of the surety members of the C-suite want before exploring GenAI or predictive AI for their operations. “The only way to actually be confident in running them effectively and efficiently with data privacy is utilizing these on-device AI chips,” Hayden says. “You’re not going to be able to enable the use cases with the cloud, so from the vertical perspective, it’s going to become increasingly nonnegotiable.”
About the Author(s)
You May Also Like