Aligning Cultural and Technical Maturity in Data Science
Here are some practical tips for organizations to leverage the full potential of data science and drive value.
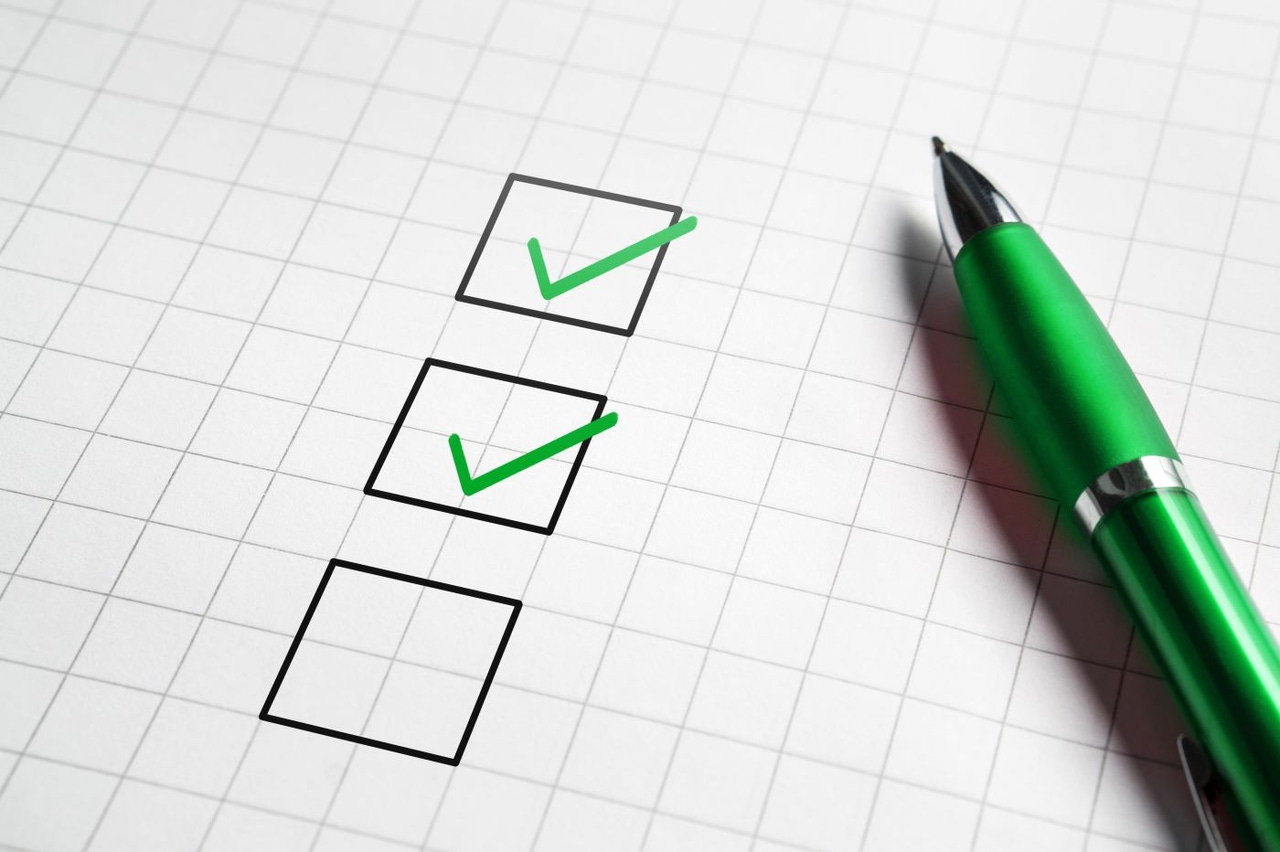
The demand for advanced analytics and data science capabilities is skyrocketing as organizations seek descriptive insights to understand what is happening and predictive insights to anticipate future developments.
However, organizations find themselves at different points on the spectrum of technical maturity and cultural readiness to fully use these capabilities. Understanding where your organization stands is the first step toward harnessing the full potential of data science.
Assessing Data Science Maturity
The journey begins with a thorough assessment of your company’s data science maturity. This involves evaluating both technical capabilities and cultural readiness. While some organizations boast high technical maturity with sophisticated data science teams, they may struggle with adoption across their organization. Conversely, others may have a strong cultural inclination towards data-driven decision-making but lack the technical infrastructure to support it.
For organizations that are culturally ready to integrate data science into their business but are technically nascent -- referred to as “aspiring” -- there are practical steps to build a robust data science presence. The key is to start small, focusing on foundational skills and gradually tackling more complex problems as the team matures.
Practical Tactics for Aspiring Organizations
Start with the basics: Begin by embedding data science teams directly within business units. This ensures that the work is aligned with business needs from the outset.
Focus on simplicity: Resist the temptation to dive into complex methodologies or advanced AI tools unless they are truly warranted. Often, simpler approaches can yield high-quality results, especially when the foundational data is robust.
Build a strong foundation: Ensure that your data is clean and well-organized. The adage “garbage in, garbage out” holds true; a solid data foundation is crucial for any data science initiative.
Find clear business problems: Define clear business problems and ensure that the complexity of the data science approach matches the problems’ scope. This alignment prevents wasted effort and resources.
Develop Foundational Methodologies
One effective strategy for embedding data science teams within the business is to ensure you prioritize a solid methodological foundation. You can then bring those methodologies to life with the use of technical packages. These are blocks of code or algorithms that can be reused across the organization. They ensure consistency in methodology and save time by preventing data scientists from reinventing the wheel. Packages can be developed by data scientists with a focus on methodology and used by those with varying skill sets, ensuring a consistent and efficient approach.
Utilizing AI to Scale and Upskill
While it’s important not to jump into new technologies like AI without a clear purpose, AI can be a valuable tool for scaling and upskilling data science teams. AI tools can assist teams by allowing them to code in unfamiliar languages, perform quality assurance, and explain code. In-house AI tools can be fine-tuned using your company’s proprietary data and can be built in such a way that neither the prompt nor response will leave your company’s network.
These tools are not perfect and are only starting to serve non-technical users, but they can address many pain points for organizations ramping up their data science capabilities, especially lean teams or teams made up of data scientists with diverse backgrounds spanning multiple coding languages.
Addressing Untapped Potential
A common scenario we encounter is the presence of untapped potential. Here, organizations have high technical maturity but low cultural adoption. This often manifests as technically proficient data science teams that operate in silos, disconnected from the broader business objectives. The challenge is to integrate these teams into the business fabric, ensuring their work drives tangible value.
This involves:
Setting business context: Ensure that data science teams understand the business context and objectives. This alignment sets them up for success.
Embedding teams: Align and embed data science teams directly with the business units they support. This fosters collaboration and ensures that data science initiatives are relevant and impactful.
Cross-functional teams: Consider forming cross-functional teams to facilitate critical connections between data scientists and business stakeholders.
Business and Technical Responsibilities
By aligning data science teams with both business and technical responsibilities, organizations can bridge the gap between cultural readiness and technical maturity, unlocking the full potential of their data science capabilities and adhering with the organization’s governance processes.
Business Side Responsibilities:
Client consultancy: Understanding the business, its use cases and challenges
Problem framing and scoping, and learning plan development
Product innovation through pilots and proof of concepts
Collaboration with account managers, engineers and product teams
Technical Responsibilities:
Science and methodology research and development
Coding and package development
Adherence to data science best practices
Learning and development initiatives
In conclusion, assessing your organization's data science maturity and addressing the mismatch between cultural and technical readiness are crucial steps toward leveraging data science effectively. By embedding data science teams within the business, creating solid data science foundations, methodologies and leveraging AI tools when it is appropriate, organizations can build a robust data science presence that drives real value.
About the Author
You May Also Like