4 Top Data Management Challenges (And How to Conquer Them)
Nobody ever said data management was easy. Fortunately, even the most daunting obstacles can be resolved by taking the right approaches.
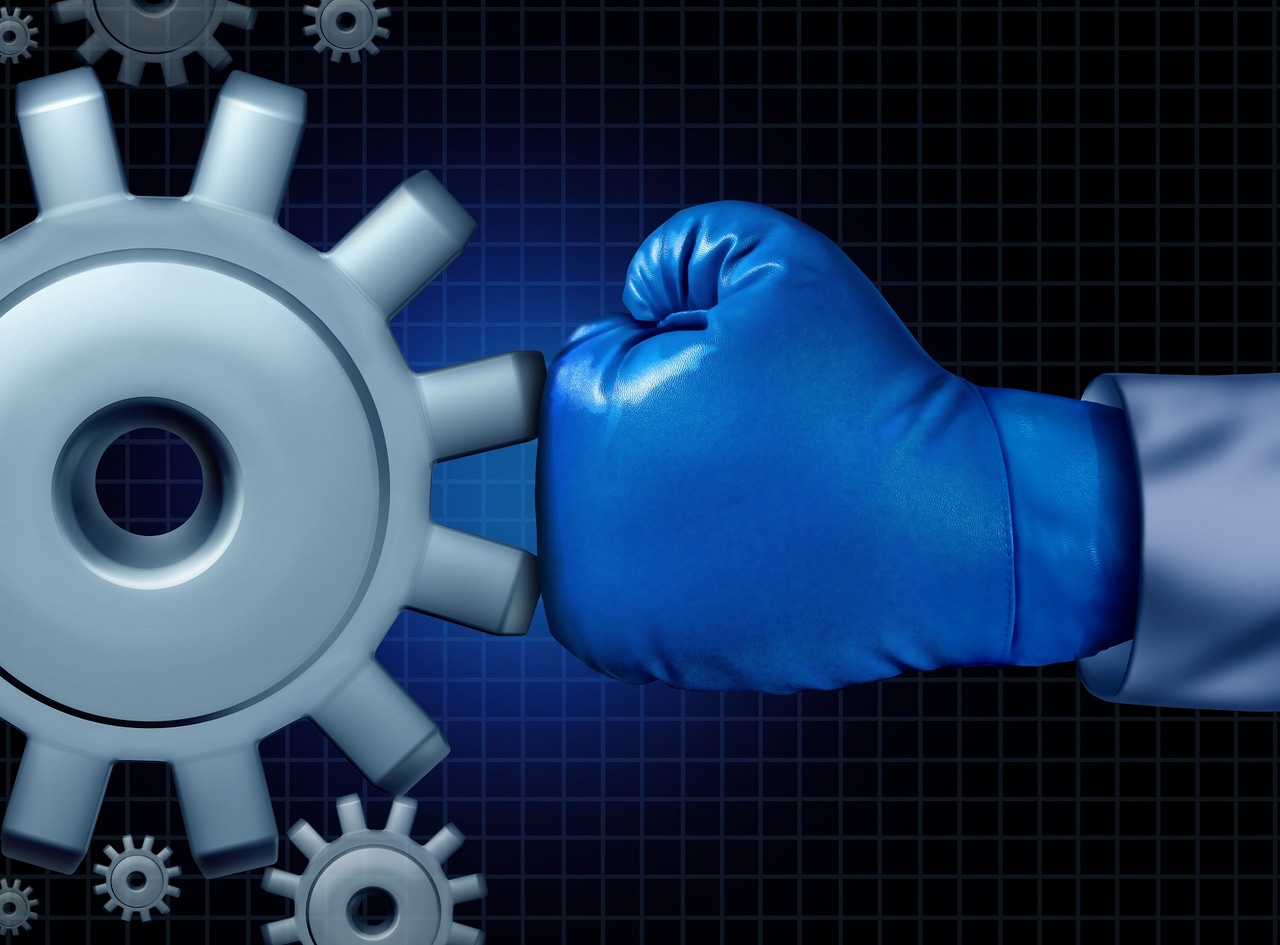
Data management includes collecting, protecting, organizing, and storing organization data, allowing it to be analyzed to create informed business decisions. Unfortunately, as more data is collected, management becomes an increasingly challenging and time-consuming task. But don’t lose hope. It’s possible to overcome even the biggest management challenges, as long as you know what to do.
The following four insights, conducted via email interviews, should help you effectively handle today’s most daunting data management tasks.
1. Data silos
Data tends to arrive from various sources and can be difficult for cross-functional teams to fully access. “This means that team members often have an incomplete picture of the effectiveness of current processes or strategies,” says Daragh Mahon, CIO at truckload carrier and logistics provider Werner Enterprises. “It’s therefore important to isolate the correct data and make it actionable to pull insights, make decisions, and pivot tactics, if needed.”
The biggest obstacle to overcoming data siloes is finding the right solution for storing large amounts of data while allowing easy access and use, Mahon says. He notes that making data readily available to the people who need it requires ample storage resources that are accessible to different team members in a form that supports collaboration, visualization and knowledge sharing.
The best way to resolve data silo issues and improve data analysis is by adopting a cloud-first, cloud-now strategy, Mahon says. “By hosting all relevant data in the cloud, companies can capture and store data and leverage AI and machine learning technology for quick analysis to inform decision-making.”
2. Data complexity
Many organizations are saddled with massive data schemas packed with thousands of tables, each containing hundreds of columns that may or may not be named in human-understandable terms. “This poses challenges when data engineers want to write new SQL queries to retrieve data -- they don’t know what tables to access or what columns to reference,” says Susan Davidson, a professor at the University of Pennsylvania’s engineering school.
As it turns out, generative AI happens to be very good at writing SQL queries from English-language descriptions of the task, Davidson notes. On the downside, generative AI tends to fail miserably when the schema is very large. “Retrieval augmented generation (RAG) is a promising avenue, and there’s active research in how to use this to improve the task query writing over extremely large data schemas.”
3. Data overload
For years, organizations have been advised to collect as much data as possible in the event it may someday prove to be valuable. Unfortunately, this has often led to the accumulation of massive amounts of structured and unstructured data lacking any underlying strategy for naming conventions, locations, or data governance. Now, many IT leaders looking at mounting storage bills with no clue as to which data is useful and valuable and which is rubbish, says Ryan Ries, chief data science strategist at cloud service provider Mission Cloud. “Often, the people who set up the system have left the company, and it’s really unclear what’s going on,” he observes. Now, the IT team has to go through terabytes of data to try and understand which data has value, which doesn’t, and build management strategies.
It’s important to dig deep to understand your data and the goals for its use, Ries says. “The challenge can be daunting, however, when there’s a ton of data to sift through.” Many organizations simply don’t have the resources or time available to sift through mounds of data and understand its value. “It’s like cleaning out your garage,” he states.
The best way to address this situation is with a formal data management strategy that specifies which types of data to retain and reject.
4. Poor quality data
Poor data quality takes many forms, including inaccuracies, inconsistencies, redundancy, and missing data. Any of these issues can undermine data management.
Data quality issues can be both costly and potentially harmful. They can render efforts in other data management areas largely ineffective. The foundation for successful data management lies in high-quality, consistent, accurate, and comprehensive data at both the content level as well as at a metadata level. Only organizations with their data quality house in order can expect the other subcategories of data management to effectively function and provide value.
Determining whether an organization’s data is both usable and trustworthy presents a significant challenge. Data, with its inherent diversity in shape, size, and structure, requires a comprehensive effort, says Bob Brauer, founder and CEO of Interzoid, a data usability consultancy. He notes that complexity can be compounded by limited control over sources as data flows in from multiple people, organizations, and processes. “Combined with natural variations in language, culture, and alphanumeric data, taming data becomes a significant and seemingly unending challenge.”
A commitment to ensuring data quality begins with making quality a key strategic goal. “An effective approach involves appointing executive leaders responsible for data quality and equipping them with the necessary budget and resources to succeed,” Brauer advises. “Key actions should include conducting comprehensive data assessments, establishing data governance strategies and rules, focusing on the most critical data areas to get some early wins, and setting measurable metrics and goals to track and manage progress over time.”
About the Author
You May Also Like