Think through your customer and supplier relationships, as well as your operational efficiency to identify the best areas for artificial intelligence.
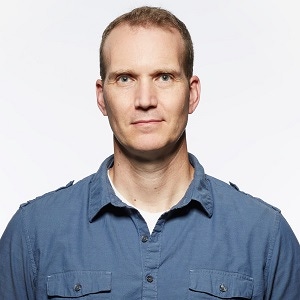
Like many organizations, your company may be pouring resources into the development of artificial intelligence. Investment in the space is skyrocketing. IDC predicts that by the end of 2018, companies will have spent 54% more on AI than they did in 2017.
However, for many, that investment has yet to pay off. A recent survey from MIT Sloan Management Review and Boston Consulting Group found that less than 5% of organizations have incorporated AI extensively into their processes. That’s no surprise, given how difficult a successful AI implementation can be. Creating and iterating on a solution, adapting your business processes to utilize it, getting buy-in from employees; each step in the process is challenging, and any mistake can be costly.
On top of that, many executives don’t understand what AI really is in a business context. When businesses talk about deploying AI today, they’re usually talking about building mathematical models that can be trained on data to make decisions. That’s a specific subset of AI called machine learning, and it’s far from the sort of generalized intelligence the term “AI” implies. Each machine learning model is specialized to make a certain set of decisions based on certain sets of data.
If you’re going to invest the resources necessary to make your models a success, you want to make sure that investment is going where it’ll have the most impact. Today’s leaders didn’t start by deploying models willy-nilly. They started where they knew machine learning could make the biggest difference.
Analysts usually apply the Five Forces concept to determine the overall competitiveness of an industry. However, that can also be a guide for businesses looking for where to test out model-driven solutions. By examining the competitive forces at play in your industry, you can decide which competitive “levers” are most likely to increase profitability, and how you can deploy models to help.
Create differentiation
The bargaining power of buyers tends to be high in industries where products are undifferentiated and switching costs are low. Companies can deploy machine learning models to create differentiation and also make changing loyalties inconvenient, decreasing the bargaining power of buyers.
Video streaming is a clear example of an industry where bargaining power of buyers is high. It is so easy to switch from service to service that many platforms lose 50% of their subscribers each year. However, industry leaders like Netflix stand out with churn rates in the single digits. That’s because they deploy models to surface the right content at the right time in the right format to keep users engaged.
Once one of these powerful recommendation engines knows a customer’s taste well, it is extraordinarily difficult for a competitor to offer a comparable customer experience. This gives services like Netflix a competitive moat that will be difficult for other companies to breach, even in a supposedly highly competitive industry.
Google controls over one-third of the total digital ad market in the US. That makes them enormously powerful suppliers in the digital marketing space. Brands that want to reach large audiences have no choice but to spend heavily on high-traffic keywords, lining the tech giant’s overstuffed pockets.
AI may not be able to break Google and Facebook’s online ad monopoly, but they can give users back some power. For example, many companies deploy automated bidding models to optimize their Google Ads spend. By taking user demographics and other factors into account, they can make sure that their ads target the customers most likely to convert. That may mean eschewing high-traffic keywords in favor of cheaper terms that offer more bang for the buck.
In the long term, companies also can leverage the dividends of their model-driven competitive advantage to right the power balance with suppliers. For example, thanks to Amazon’s massive success in the retail industry, at least 21 public companies now generate 10% or more of their revenue through the e-commerce giant. That gives Amazon significant power over its suppliers, which it recently applied to shift more of its delivery expenses and other costs onto them.
Innovate to counter the threat of new entrants
If barriers to entry in a given industry are low, machine learning models can help incumbents stay competitive with upstarts. Those models can bring costs down by enabling automation, eroding a key advantage of young, lean startups. Models can also be the core of new products that replicate or even improve upon the innovative options upstarts offer. This is an area where legacy companies actually hold an advantage, having years’ worth of rich data on their customers that a newer firm would not have, and upon which a finely tuned model could be built.
For example, you might think of the highly regulated, capital-intensive insurance industry as a sector with a high barrier to entry. However, a number of new startups have entered the field with innovative products underwritten by traditional insurers or reinsurers. Incumbent insurer Allstate has stayed ahead of the game with new model-driven services like its QuikFoto claim app, which uses machine learning to instantly assess minor accident damage from user photos. While some startups like Cover offer similar services, Allstate is able to leverage its vast trove of customer data as well as its staff’s data science expertise to create a more sophisticated solution.
Raise switching costs
A substitute is a product or service from another industry that could replace what your company offers. Companies can deploy machine learning models to raise switching costs between substitutes just like they can use it to raise switching costs between different brands within an industry. For example, the threat of substitutes poses a challenge for the cruise industry, because almost any other vacation could be a substitute for a cruise. Customers planning a getaway might opt to stay at an all-inclusive resort, visit Disney World, or go camping instead.
Carnival Cruise Lines reduces the threat of substitutes by using machine learning models to give its guests a one-of-a-kind personalized experience. On its Princess cruise lines, it distributes a device called the Ocean Medallion to passengers. The Medallion can be used to open doors and order drinks, but more importantly, it tracks each passenger’s location, what purchases they make, and what activities they sign up for. Over time, the system “learns” that passenger’s individual preferences, and starts to tailor offers specifically to them.
For customers who have sailed with Carnival in the past, opting for a different vacation now comes with a cost: the loss of personalization. Yes, visiting an all-inclusive resort might be a nice change of pace. However, the staff there won’t remember you prefer attending live shows to going out dancing at night, or that you like pina coladas better than daiquiris. Strategies like these can be deployed across industries to reduce the threat of substitutes.
Drive down costs
In an industry where rivalry is intense, machine learning models’ ability to drive cost savings becomes especially important. Under pressure to cut prices, firms will compete away their profits unless they can find ways to save. One way model-driven companies pad profit margins is by using machine learning to optimize their supply chains. (Amazon is masterful at this, and in their current price war with Walmart, are ratcheting up the pressure further.) There’s enormous opportunity across industries here: Only 13% of companies report that logistics is the division of their organization leading AI adoption, according to CIO.
Whether it’s by cutting expenditures, introducing differentiation, or raising switching costs, harnessing the power of machine learning models can yield enormous dividends for any business. However, the road to successful implementation can be long and hard. Before you start, you want to be sure you’re deploying machine learning models where they make the maximum impact for your business. By studying the competitive forces at work in your industry, you can determine where machine learning could help your organization gain an edge.
Josh Poduska is Chief Data Scientist at Domino Data Lab.
About the Author(s)
You May Also Like