Big Data Projects: 6 Ways To Start Smart
Don't let poor definitions, lack of best practices, or uncertainty about goals sidetrack your next big data project.
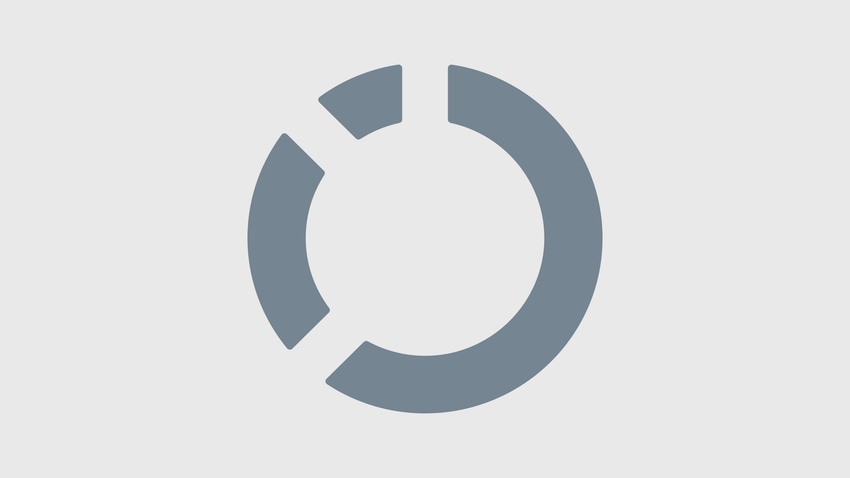
There are three solid rules describing how to successfully introduce big data into an organization. Unfortunately, to paraphrase W. Somerset Maugham, no one knows what they are.
In literary fiction, that's not a terrible drawback. At least it wasn't for Maugham, who wrote classics such as Of Human Bondage and The Razor's Edge, apparently without knowing the performance, design, or user-experience requirements for either project.
Big data projects require more precision if they're to succeed in delivering analytic tools or frameworks with enough power to handle the volume, variety, and timeliness required to qualify as "big" data.
One problem facing organizations is the lack of consistent, useful best practice guides that define issues common to most organizations, according to Mike Boyarski, director of product marketing for business intelligence/big data software vendor Jaspersoft.
As a category of business data analysis, big data is so new, so ill-defined, and the ecosystem of tools so immature that the long list of best practice documents published by big data vendors offers few consistent recommendations.
"There seems to be a lot of uncertainty about the value proposition, the ROI of big data, and about the tools that would let people take advantage of it," Boyarski said, citing a survey of prospective big data managers and developers that Jaspersoft will release Tuesday.
[ Get more more advice on avoiding bit data pitfalls and risks. See 10 Big Data Migration Mistakes. ]
End user organizations are a lot farther along in the development of their own big data implementations than Boyarski expected, even as respondents complained they lack sufficient guidance to be comfortable with their own project implementation plans.
As was the case with cloud computing, business unit managers seem to be pushing big data through the project pipeline as they anticipate the potential benefit of more complete, more insightful analysis of customer behavior than they've had until now, according to a survey from market researcher CSO Insights, which specializes in analyzing the effectiveness of corporate sales efforts.
Only 16% of organizations responding to the survey have any big data capabilities, but 71% of managers expect that adding one would have a significant positive impact on sales, the survey showed.
Despite significant, sustained demand for its reputed capabilities, the market for big data analytics is so fragmented and filled with small players that vendors are still trying to work out their own strategies and positioning according to IDC analyst Dan Vesset.
Companies that produce or collect non-traditional data--social networks or Web behavioral data collection and analysis companies, for example--step on the toes of traditional BI and database companies, who are still considering whether to get into the data collection business, according to Vesset.
There are some consistent elements that have to be taken into account or changed to support a broader analytic mission, however, according to Ash Ashutosh, CEO of data management vendor Actifio.
At their most basic, the challenge of moving into big data begins with the process of storing, processing, and managing the new data. Cloud computing platforms, storage area networks, and other scale-out systems can deal with big data storage demands; servers installed as purpose-built data processors can help avoid bottlenecks, according to Ashutosh.
Consider these six steps before you start your next big data project.
1. Identify missing pieces, whether they be tools or data.
The big gap is in tools designed to collect, deduplicate, tag, and process new types of metadata, and that give big data the context and meaning that make it valuable, according an IDC report on big data migrations published in June 2011.
Databases of text messages, asset-management information, and other content generated by users with smartphones is made vastly more useful with the addition of location data, but few analysis or data management tools are equipped to collect data from smartphone GPS chips or combine it with existing data or databases so it can be analyzed coherently, the report said. 2. Understand the data you have and the data you need.
Another major complicating factor is the need to audit and report on available data types before even approaching end users to collect project requirements--a reversal of the traditional development process, according to Krish Krishnan of consultancy Sixth Sense Advisors.
3. Know what you're trying to accomplish.
Creating clear, easy-to-understand business requirements is a critical next step, according to Krishnan. Without clear requirements it's impossible to map out the timeline and specific steps to complete the project, let alone the skills and training required to make it useful for employees, said Krishnan, who co-wrote Building the Unstructured Data Warehouse, a guide to building big data systems published in January 2011, before the term "big data" came into vogue.
4. Find and hire data scientists .
Absolutely critical to the success of any big data project is the ability to define, understand, manage, and contextualize data from many sources in many formats--a challenge that falls under the expertise of a job description alien to most IT departments, and even most corporations: the data scientist.
Forty-five percent of BI projects fail due to a lack of data expertise on staff, according to an April survey from GigaOm. Big data expertise requirements are more rigorous than most BI projects, meaning failure rates due to a lack of expertise should be even higher.
According to IBM's definition, data science requires training in computer science, applications, data modeling, statistics, analytics, and advanced mathematics. It also requires training in business processes or business management in order to identify requirements of the organization and the data that could match them.
"A data scientist does not simply collect and report on data, but also looks at it from many angles, determines what it means, then recommends ways to apply the data," IBM's summary said.
Data scientists fall into the same job category (and sometimes job description) as business analysts, data analysts, and specialists in analytics, but tend to be more specialized, have more experience and be better educated than data specialists who don't hold the title, according to a survey of data-analytic specialists (free registration required) published by BI vendor SiSense Aug. 7.
Only 5% of most data professionals hold a PhD in a relevant specialty, for example, while 35% of data scientists hold one, the survey showed. Data scientists also make more money than other data professionals. Those without management titles averaged between $70,000 and $90,000 per year, compared to $65,000 to $70,000 for more traditional data specialists.
5. Understand speed, manage expectations.
A critical element in big data is rapid acquisition and analysis of data--characteristics that are rare in any IT system described with the word "big," according to Chad Richeson, CO of BI consultancy Society Consulting.
Big data systems need to be easily and quickly adaptable, rather than being constrained by the 18- to 24-month development cycles typical of most data projects, Richeson wrote. It should be possible to make changes to the type or source of data in three- to six-month cycles, for example.
To keep big data analyses relevant, the data that goes into them should be fed into the analytic system at the same time it hits the production system, or immediately afterward, Richeson wrote.
To accomplish those things, big data project managers have to become good at prototyping new systems or changes to existing systems, and establish processes to sample data as it is collected and verify its quality.
Without data of unquestioned quality, no analysis, no matter how complete, is likely to be accurate, relevant, or useful to the end users who are supposed to benefit from the system, according to Richeson.
6. Check with end users.
The last major step is one that has to be taken at the beginning of a big data project and repeated throughout implementation and beyond, according to all the experts cited here: Big data projects have to answer questions that help specific business unit employees do their jobs.
Slick analytics are great, according to JasperSoft's Boyarski, but they're no match for simple, direct answers to questions that have real impact with end users.
Without rigorous, regular input from end users on what data are relevant and which answers are useful, no big data project, no matter how cunningly designed or effectively implemented, will deliver the kind of results it needs to succeed.
Cloud services can play a role in any BC/DR plan. Yet just 23% of 414 business technology pros responding to our 2011 Business Continuity/Disaster Recovery Survey use services as part of their application and data resiliency strategies, even though half (correctly) say it would reduce overall recovery times. Our The Cloud's Role In BC/DR report shows how the combination of cloud backup and IaaS offerings can be a beneficial part of a "DR 2.0" plan. (Free registration required.)
Read more about:
2012About the Author(s)
You May Also Like
How to Amplify DevOps with DevSecOps
May 22, 2024Generative AI: Use Cases and Risks in 2024
May 29, 2024Smart Service Management
June 4, 2024