Seeking an Oasis in a Data DesertSeeking an Oasis in a Data Desert
Gaps in data quality, particularly due to supply chain issues during the pandemic, is becoming a serious influence on planning effective machine learning models.
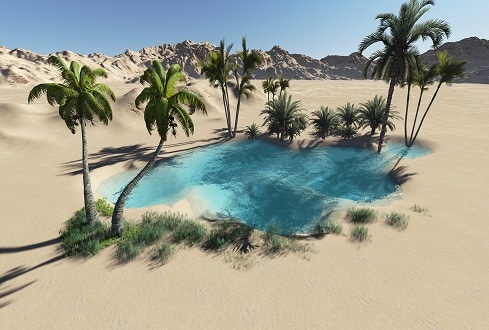
When it comes to weather, we treat barometers as good indicators of pressure changes that predict potential rain. We trust these indicators are reliable because the input information is not influenced by human activity.
The same cannot be said about search engines. They are reliable for discovery of informative media. But as discussion regarding instances of misinformation and error management in machine learning grows, technologists must think about how gaps in search engine queries impact our algorithms and ultimately our world. Data voids encapsulate those gaps.
Data voids are query differences between the quality of what people receive in a query and available authoritative information used in the query. The gap is a byproduct of how information delivery on the web evolved. Filling information gaps has typically involved commercial purposes, but over time the internet incorporated more unvetted sources in noncommercial media and as a result spread misinformation on social and political topics.
Michael Golebiewski and danah boyd of Microsoft first coined the phrase data void in a 2018 report, Data Voids: Where Missing Data Can Easily Be Exploited. Boyd has made several presentations educating the public about real world concerns that data voids have introduced so far.
To better imagine how this gap evolved, think about The Long Tail theory, the statistics concept that Chris Anderson advocated as a new business approach. The theory, that smaller volumes of items could be sold more profitably online, herald the internet as a commerce platform for new products and services. But over time the world adopted the internet as a resource for more than retail products. The long tail has morphed to include the extension of noncommercial topics that may not be in high demand and updated frequently yet have been infiltrated with speculative ideas treated as an absolute truth among unsuspecting citizens. The impact is especially felt in social and political topics.
Because people rely on search, data voids open the door to people being manipulated on many societal issues. Engine queries that return too little information or no results breed an opportunity for manipulators to fill in these gaps with their own information. Manipulators build an ecosystem around strategic new terms related to the low-search-volume queries. They then try to pass those terms into mainstream media. Boyd highlighted Frank Luntz as an example in her 2019 presentation. Luntz taught members of the Republican party how to insert strategic terms into news so that journalists would inadvertently mainstream the terms and amplify a desired message, shaping the cultural acceptance of information at the expense of truth.
The use of strategic terms exacerbates the spread of misinformation online. Data void topics associated with social and political issues are ripe targets for manipulation. Conspiracy theories thrive on a portion of information taken from current news or general knowledge. People share this information through posts and memes. With many actors using the internet to be speculative, the effort can creep into the information of other cultural and media institutions. While debates may help combat misinformation on a one-to-one scale, they do not counter the scaling up of harassment, manipulation, or even worst, mass public action. The January 6th attack on the US Capitol is the epitome of how messaging can grossly mislead the public.
The impact of data voids can go beyond misleading search results. Social media data along with search data are often included with semantic analysis that relies on machine learning to support solutions to societal issues such as mental health and racial discrimination. For example, Professor Luo at the University of Rochester created a research study on how mental health during the COVID-19 pandemic is expressed through tweets on Twitter. Studying sentiment analysis on a broad body of text aids the fight against policies based on data generalizations that have the same chilling impact as legislation that enacts societal discrimination or initiates civic projects that enhances gentrification or gaps in distributing vaccinations.
Within organizations, operations teams must be vigilant about how data from online sources such as search and social media are judged against their specifications within a data model. Teams must conduct algorithmic audits to inspect the fidelity of the data. They can do so through observability, processes meant to provide a deep understanding across different stages of a model development cycle. This sets up alerts that protects downstream systems from being corrupted with misinformation from data voids. It will also align team workflow to address the kind of data voids that lead a chatbot astray, like the notorious racially charged text manipulation of Microsoft's Tay chatbot, or for an algorithmic model to overlook redlining concerns, like those raised in the Bloomberg report on Amazon's Prime rollout back in 2016, which noted how Prime was not offered to urban Black neighborhoods.
In these days of machine learning, gaps in data quality are a glaring problem to any enterprise. The world is operating in an economy guided by data. Tech often guides us to solutions before we need guidance, making life easier. But guidance based on manipulated information because of data voids opens a door for misguided technological choices, bad decisions, and misguided people. Manipulation and misinformation from data void hits with a pervasive force like any other destructive storm.
Related Content:
What Tech Jargon Reveals about Bias in the Industry
Data Bias in Machine Learning: Implications for Social Justice
What Do We Do About Racist Machines?
About the Author
You May Also Like