How to Democratize Machine Learning Across the EnterpriseHow to Democratize Machine Learning Across the Enterprise
Business leaders are asking how they can scale data science and ML capabilities to improve the bottom line. Learn how Capital One has democratized machine learning across the enterprise for non-technical users.
August 1, 2022
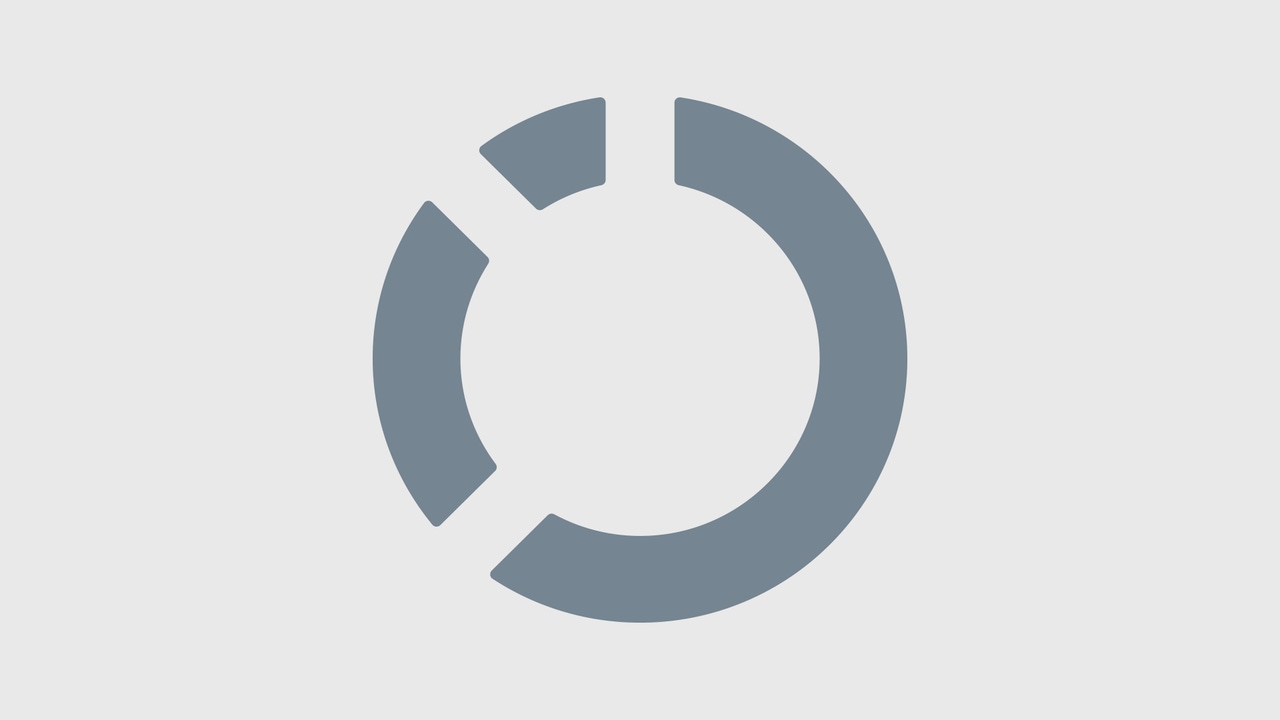
Sponsored by Capital One
(Sponsored Article)
Prior to machine learning (ML), complex and large-scale data set analysis was conducted by statisticians. Today, organizations rely increasingly on ML to do this work with greater accuracy, speed, and scale. As more companies move to the cloud and begin managing big data, business leaders are now asking how they can scale data science and ML capabilities to improve the bottom line.
Helping to fuel the democratization of ML are data science and ML platforms that can bring this technology to a broader set of users such as business analysts. According to a 2022 Gartner CIO and Technology Executive survey, 48% of respondents have already deployed or plan to deploy AI/ML in the next 12 months. That makes these platforms a necessity for ML operations since there is a shortage of data science and ML talent at most organizations.
We’ve democratized ML at Capital One by creating an internal ML platform that provides Capital One associates with governed access to algorithms, components and infrastructure for reuse. This allows non-data science and machine learning practitioners to leverage ML for business decisioning with impactful results. An example is our use case for credit card fraud defense where we’re using home-grown and open-source ML algorithms hosted by a shared platform to detect anomalies and automatically create defenses.
Based on our learnings, here are some best practices to democratize ML across your organization, from modernizing your compute environment to standardizing tools, processes and platforms, and leveraging automation in production.
Modernize the Compute Environment
A modern compute environment leverages the instant provisioning of infrastructure and processing power provided by the cloud to positively impact every part of the model development lifecycle. This computing power at scale can enable a high-performance data ecosystem for decision support with the ability to:
Check for completeness and quality as data is brought into the system;
Enable discoverability and governed access to data for analysis and ML model development to drive meaningful insights; and
Scale models to handle large and complex datasets in parallel.
With increased processing power enabled by the cloud, complex and large-scale data set analysis is conducted more efficiently, replicated more easily, and democratized for non-technical practitioners.
Standardize Tools, Processes & Platforms
Standardizing tools, processes, and platforms allows data scientists and engineers to more easily identify, access data, and build on the foundations established to deploy ML models. Bespoke model pipelines can be inefficient and brittle, inhibiting the ability to scale and make ML accessible to non-expert practitioners. Standardization includes moving teams to the same stack, focusing on collaboration, bringing down silos and prioritizing reusable components and frameworks across all ML efforts.
Developing foundational platforms can make ML efforts adaptable, well-managed, and scalable in order to assist with virtually every aspect of developing, deploying, and maintaining models. In fact, common platforms can help and store model training and execution information, like parameters and outcomes, in a repeatable and searchable way so that models can be more easily audited and reproduced.
Advance Model Monitoring & Training
Once ML models are in production, automation can help companies achieve continuous delivery of a model prediction service. Automating ML model monitoring and training can ensure a model is performing when it’s pushed to production and help teams make better decisions about when action is required to retrain a model. This automation provides engineers with confidence in consistent reproducibility and maintenance.
Human oversight of automated model monitoring and training within an organization is critical. A centralized governing body can manage the processes, controls, monitoring, and technology infrastructure to help scale ML responsibly while facilitating greater transparency across development efforts.
Automation also improves developer experience by allowing technologists to focus on feature and model development instead of excessively onerous and manual issue resolution.
As companies begin to scale ML across the enterprise it’s important to follow best practices and support continuous learning and training. If done responsibly, ML democratization can provide a wide set of non-technical users with the ability to conduct analysis and generate insights at scale. This can provide meaningful business value across the organization, much as we’ve experienced with our ML-driven credit card fraud defenses.
Dave Kang is SVP and Head of Capital One Data Insights leading an organization of data scientists, software and ML engineers as they build solutions to democratize machine learning.
You May Also Like