Manager is checking off four workers in a virtual planning matrix to select them for a work team.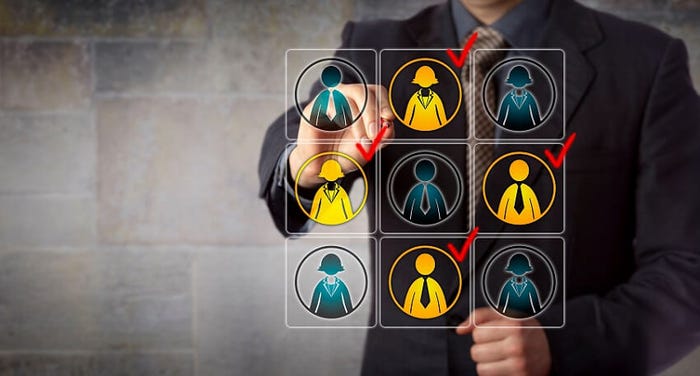
IT Leadership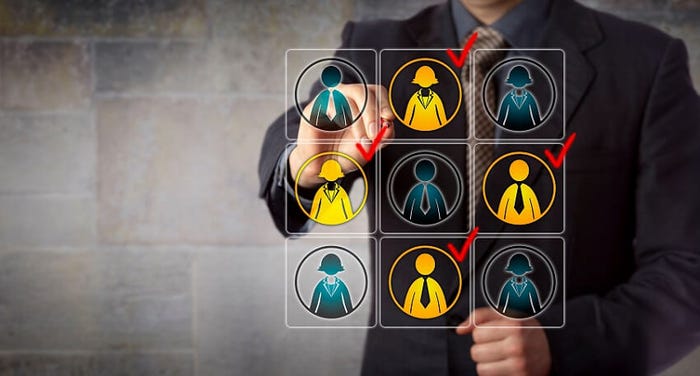
Why Your Enterprise Should Create an Internal Talent MarketplaceWhy Your Enterprise Should Create an Internal Talent Marketplace
For a growing number of businesses, talent acquisition begins at home. An internal talent marketplace can help IT leaders find skilled individuals in their own organizations.
Never Miss a Beat: Get a snapshot of the issues affecting the IT industry straight to your inbox.