Moving AI Forward: Why You Need to Slow Down Now to Scale Later
Companies looking to truly take advantage of AI’s full potential must first address the most common problems that again and again hold the industry back.
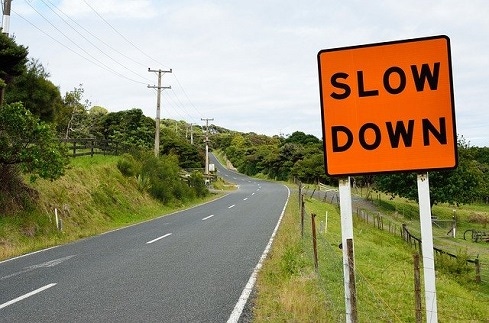
A lot of companies had big plans for artificial intelligence (AI) last year. In 2020, however, those ambitions have gotten a little bit smaller. In an annual survey my company conducts, only 4% of business and technology executives who work with AI said that they planned to roll it out across their organizations in 2020. A year ago, 20% had said the same.
That’s a big drop, but I can’t say that I’m surprised. There are many reasons behind this retrenchment, but three issues come up again and again in the AI work I do with organizations across the globe. Fortunately, they all have solutions.
1. Lack of labeled data
In 2020, we’ll see a focus on what we call “boring AI,” using it to streamline processes or to solve universal pain points, such as extracting data from forms. In our survey, executives ranked using AI to operate more efficiently (44%) and to increase productivity (42%) as the top benefits they expect from the technology in the coming year.
To get the job done, however, AI needs data that -- among other things -- is accurate, unbiased, secure, and labeled. But many business leaders, even as they pushed ahead with AI initiatives, haven’t focused enough on their data. Just 13% said that standardizing, labeling, and cleansing data for use in AI systems was a top priority for them in the coming year.
It’s understandable why they’d avoid it: Labeling data is resource intensive and it requires domain expertise, and usually people outside of the AI organization. In pharmaceuticals, for example, we built an AI application for adverse drug interaction and the system required a clinical expert to label different drug reactions as high, medium or low risk.
One effective strategy for overcoming the labeling challenge is to use AI to help you do it. You start with machine teaching, where the business specialist “tells” or “shows” the AI how to label data. Then you incorporate active learning, where AI, following what it has learned from the human experts, starts to perform the labeling itself, but with a human supervising and correcting it.
Over time, AI both makes fewer mistakes and learns more quickly from those mistakes. Labeling becomes more efficient and both human and AI keep learning together -- what we call agile learning.
2. Overlooking the need for bilinguals
Business leaders know AI talent is still scarce and they’re attacking the problem on two fronts: hiring and upskilling. Forty-six percent said they’re rolling out AI upskilling, 38% are implementing credentialing programs for data scientists and more advanced AI skills. More than a third are also exploring partnerships with community colleges and universities. Such relationships are important and reflect a shift in the AI talent landscape: In 2018, more than twice as many AI PhD graduates went into industry positions versus academic jobs, according to the 2019 AI Index, which was recently released (and to which I and other PwC colleagues contributed).
But the challenge doesn’t stop there. You need to ensure that AI specialists are put to work on the right business problems, and that they can productively collaborate with others in the organization. What you really need are bilingual employees: data scientists who have some notion of the business and domain experts who understand what problems AI can solve and what solutions might look like. You also need them to be able to easily work together.
One way to do that is by building a digital platform where everyone can collaborate, identifying the problems that call for AI and beginning to test and learn the right approach. You also must give people the time and incentives to do so. And you must make AI skills an everyday part of working, by incorporating workbenches and tool kits into their work and decision flow.
Even your techies will have to learn more than one “language.” A critical AI capability is machine learning operations (MLOps), which combines expertise in data science with software engineering and IT operations. That’s essential for operationalizing AI so that it is integrated with enterprise systems such as CRM, general ledger, and procurement, working 24/7 as part of key functional areas. To have enough MLOps engineers to keep everything up and running, companies will have to develop them organically, through incentives and platforms for upskilling.
3. Lack of return on investment
The other barrier I see is around return on investment (ROI). Likewise, in our survey, executives cited it as the biggest challenge, followed by other challenges that were also less about the technology and more about how business operates.
Proving ROI is hard because AI usually delivers value indirectly, by helping employees and other technologies work better. It also often works best as one of several moving parts in an integrated package. Your AI investment may, for example, help business leaders make better choices and improve employee engagement by freeing them from tedious tasks. But there is often no baseline to compare ROI against. There’s no standard for time it takes to complete a specific task, such as analyzing potential drug interactions in the prior example.
So, can you prove that your team made a better decision because of AI? Can you quantify the value of employees spending less time crunching numbers and more time figuring out how to grow the business?
The answer is, yes. But not with traditional metrics. You need new ones that measure efficiency, effectiveness, and innovation. For example, once you have that centralized platform in place where employees can access AI tools and services, you might measure an increase in its use. Or, as employees are upskilled and begin learning by doing, you might measure the increased use in common tools or models, such as for extracting data or converting speech to text.
Moving forward with a responsible AI approach
As business leaders tackle these issues, they’ll need to maintain a steadfast focus on mitigating AI risk. Only about one-third of respondents to our survey reported having fully tackled risks in critical areas such as data, AI models, outputs, and reporting. The solution here is to make AI responsible, integrating the enterprise-wide processes, tools, and controls needed to address critical areas like bias, explain-ability, cybersecurity, and ethics.
That’s essential as companies recalibrate their AI ambitions. And if they are carefully rolling out AI where it can solve practical problems (and achieve measurable ROI) right now, while laying the foundations to take AI enterprise-wide soon after, this current retrenchment won’t even be a bump in the road. It will be a launching pad into an AI-powered future.
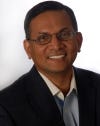
Anand Rao is Global & US Artificial Intelligence and US Data & Analytics Leader at PwC, with over 24 years of industry and consulting experience, helping senior executives to structure, solve and manage critical issues facing their organizations. He has worked extensively on business, technology, and analytics issues across a wide range of industry sectors including financial services, healthcare, telecommunications, aerospace & defense, across US, Europe, Asia and Australia.
About the Author(s)
You May Also Like