Kicking Off A Data Science Project: 4 To-DosKicking Off A Data Science Project: 4 To-Dos
Be properly prepared before diving into a data mining expedition, veteran data scientist Carla Gentry advises.
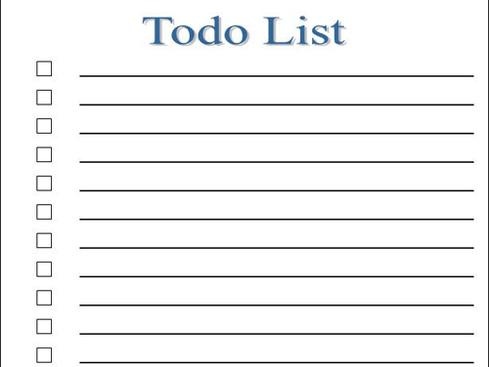
10 Lavish Monuments To Tech Egos
10 Lavish Monuments To Tech Egos (Click image for larger view.)
Veteran data scientist Carla Gentry has accrued one of the prime benefits of experience: She has made enough mistakes to know how to avoid making them again.
Gentry, who runs the consultancy Analytical Solution, has plenty to say about data science projects -- including what you should do before you even get started.
1. Differentiate data science from big data.
Gentry gets the current obsession with data science, given the hype around big data. Yet while big data may be a relatively new phenomenon, data science is not.
"Data science has been around for decades, and it’s not just big data. I hear a lot of people clumping these two together like they go hand-in-hand, which I agree with to an extent," Gentry said via an email interview. "However, big data needs data science, but data science doesn’t necessarily need big data. Most of the data a typical company handles on a daily basis or houses internally is not big data."
[Still assembling your team? Read How To Build A Successful Data Science Team.]
Although big data might be most obviously linked to big organizations, data science can be useful to companies of all sizes and with all manner of datasets. "Even Facebook and Google break up or segment their data into workable pieces. Data science is big, small, structured, unstructured, messy, clean, et cetera," Gentry says.
2. Learn to speak at least two "languages."
Data science "is more than just analytics. As a data scientist, you’ll become a liaison between the IT department and the C suite," Gentry says. "You have to talk both languages and you have to understand the hierarchy of data. You can’t be just an architect or data expert."
In other words: Time and money spent on a data science project might be wasted: You can't properly convey the results to the right audiences. It's not a gig for back-office number-crunchers with no ability to communicate or negotiate. Make sure you've got clear goals and the right team to put them in place before setting off. Likewise, know the stakeholders and involve them appropriately.
"What really matters in data science is the team effort and your role as a liaison. You want to be able to give insight, which requires knowledge of your audience. If your audience is the C suite of a multimillion-dollar company, you’re going to need everything you have to back up your conclusions. Be able to prove it, and be prepared for questions."
3. Know the servers, environment, and databases.
This is where those aforementioned mistakes come in: Gentry's crashed enough servers during past projects to know the importance of a thorough understanding of all the moving parts and pieces involved. (She's blogged extensively on this particular topic, too.) It's a prerequisite before the project gets going in earnest; otherwise, there's bound to be downtime and other pain along the way. How many servers are there? Will you be working during normal business hours or during slower periods? Are you working in a test environment or a live one? How are the databases joined? And so forth.
Ultimately, "I really don't care what kind of servers, environment, and databases [are involved]," Gentry said. But she stressed that a complete understanding of the infrastructure enables the data scientists to plan ahead and quickly resolve issues as they arise instead of fumbling around blindfolded. Knowing the servers enables smart decisions around load balancing or toggling, for example. Gentry also emphasizes the importance of star versus snowflake schema and putting real thought into the decision.
4. Cleanliness is next to (data) godliness.
Last, but definitely not least, Gentry noted that efficient, timely projects require clean data. If you don't bother to cleanse the data in advance, you're adding unnecessary slowdowns.
"If you took a dirty database that was not normalized and indexed, it could take hours longer to run and even get hung up and not complete at all. So you come in the next day expecting the job to be done, and it's: 'connection to database lost due to error or timed out.'
"Your company has large amounts of data, and you want to make sure your queries are correct," Gentry says. "Whatever tool you use, make sure you have your data cleansed. You want to know that it’s normalized and indexed so that things run smoother."
Engage with Oracle president Mark Hurd, Box founder Aaron Levie, UPMC CIO Dan Drawbaugh, GE Power CIO Jim Fowler, former Netflix cloud architect Adrian Cockcroft, and other leaders of the Digital Business movement at the InformationWeek Conference and Elite 100 Awards Ceremony, to be held in conjunction with Interop in Las Vegas, March 31 to April 1, 2014. See the full agenda here.
About the Author
You May Also Like